Ishikawa diagram
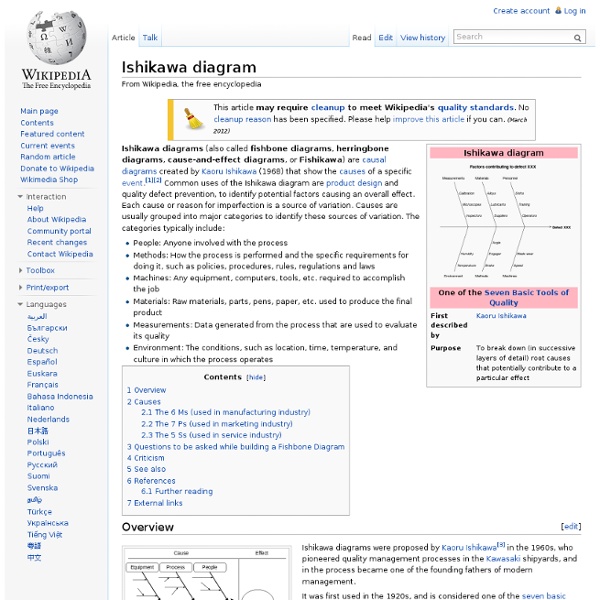
Ishikawa diagrams (also called fishbone diagrams, herringbone diagrams, cause-and-effect diagrams, or Fishikawa) are causal diagrams created by Kaoru Ishikawa (1968) that show the causes of a specific event.[1][2] Common uses of the Ishikawa diagram are product design and quality defect prevention, to identify potential factors causing an overall effect. Each cause or reason for imperfection is a source of variation. Causes are usually grouped into major categories to identify these sources of variation. The categories typically include: Overview[edit] Ishikawa diagram, in fishbone shape, showing factors of Equipment, Process, People, Materials, Environment and Management, all affecting the overall problem. Ishikawa diagrams were popularized by Kaoru Ishikawa[3] in the 1960s, who pioneered quality management processes in the Kawasaki shipyards, and in the process became one of the founding fathers of modern management. Causes[edit] Causes can be derived from brainstorming sessions.
Official Mind Mapping software by Tony Buzan
Visualizacion de Informacion - Revista
Una de las causas (que no la única) del largo silencio de esta newsletter durante el año ha sido la elaboración y puesta en marcha del primer postgrado sobre Visualización de Información del que tengamos conocimiento que se realiza en España. Hospedado en el Instituto de Educación Continua (IdEC) de la Universitat Pompeu Fabra ha sido codirigido por los Profesores Josep Blat y Juan Carlos Dürsteler y coordinado por Víctor Pascual Cid. Este postgrado surge del convencimiento, desde hace ya algunos años, de que para avanzar en la Visualización de Información había que formar a la creciente masa crítica de profesionales que cada vez más necesitan y hacen uso de técnicas de visualización para analizar y comprender los datos. Existen en diferentes lugares del mundo distintos cursos introductorios o avanzados sobre el tema, preferentemente dirigidos a profesionales de la informática (ver por ejemplo el website Infovis-wiki.net ). El Programa El desarrollo del curso Resultados
The Theory Underlying Concept Maps and How to Construct and Use Them
Concept maps are graphical tools for organizing and representing knowledge. They include concepts, usually enclosed in circles or boxes of some type, and relationships between concepts indicated by a connecting line linking two concepts. Words on the line, referred to as linking words or linking phrases, specify the relationship between the two concepts. We define concept as a perceived regularity in events or objects, or records of events or objects, designated by a label. The label for most concepts is a word, although sometimes we use symbols such as + or %, and sometimes more than one word is used. Figure 1. (click on an image for a larger view) Another characteristic of concept maps is that the concepts are represented in a hierarchical fashion with the most inclusive, most general concepts at the top of the map and the more specific, less general concepts arranged hierarchically below. Another important characteristic of concept maps is the inclusion of cross-links. Figure 2.
Related:
Related: