Datameer - Big Data and Hadoop Analytics
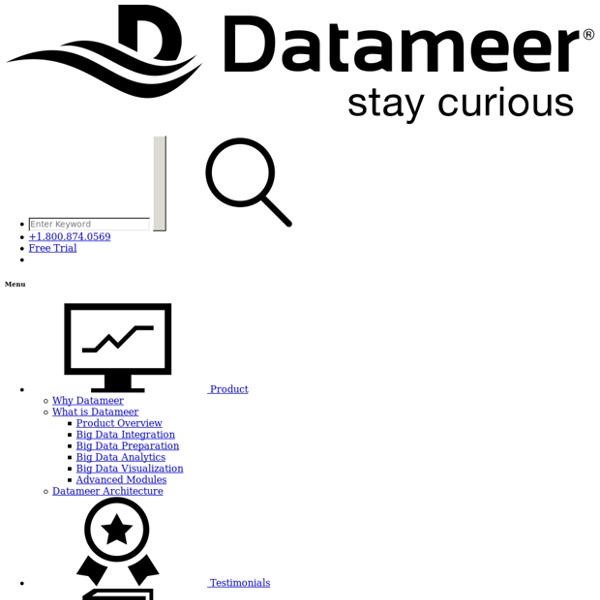
Data Visualization - Free Trial of Datameer
Datameer’s extensive library of widgets includes tables, graphs, charts, diagrams, maps, and tag clouds which enables users to create simple dashboards or beautiful visualizations. The end result, data visualizations that communicate data. Beyond static business intelligence dashboards. Get started today and share your data visually on any device. Features: Import your data Start analyzing Show off your infographics © 2014 Datameer, Inc.
5 trends that are changing how we do big data — Data | GigaOM
Big Data Analytics
vizualize.me: Visualize your resume in one click.
Why Splice | Splice Machine
Organizations are eager to tap into the wealth of data that is at their fingertips, but mere analysis is no longer sufficient. Time is a critical factor when it comes to uncovering insights that can be turned into rapid actions. This makes accessing near-real-time data an imperative for companies who are striving to realize the promise of Big Data by becoming real-time, data-driven businesses. Real-time, analytical applications are emerging to collect, analyze, and react to data without the high costs and poor scalability of traditional databases. Splice Machine, the real-time SQL-on-Hadoop database, can be the lynchpin to these applications because of its ability to Process real-time updatesUtilize standard SQLScale out on commodity hardware As a general-purpose database flexible to handle both analytical (OLAP) and operational (OLTP) workloads, Splice Machine can be used anywhere that MySQL or Oracle can be used, but with the advantage of scaling out with commodity hardware.
Основана в 2003 г., большая компания - 560
How one startup wants to inject Hadoop into your SQL — Cloud Computing News
5 low-profile startups that could change the face of big data — Cloud Computing News
Related:
Related: